AI project to use medical records to speed rare disease diagnoses
Models will look for signs, symptoms that suggest undiagnosed rare diseases
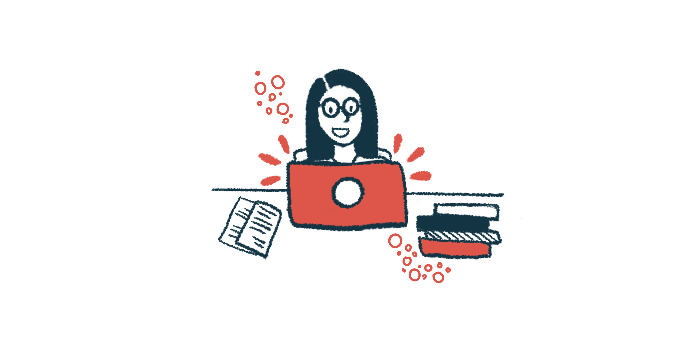
Engineers and healthcare providers at the University of Illinois are joining efforts to accelerate the diagnosis of rare diseases such as aromatic l-amino acid decarboxylase (AADC) deficiency with the help of artificial intelligence (AI).
The goal is to use machine learning models to learn from available electronic medical records, which already include clinical and demographic data, as well as physician’s notes, from thousands of people. The insights will then be used to build knowledge graphs that provide graphical relationships between data, allowing clinicians to run appropriate tests for the most relevant diagnoses.
Funded by a Jump ARCHES grant, the project will be led by Adam Cross, MD, a pediatric hospitalist and professor at the University of Illinois College of Medicine, and Jimeng Sun, PhD, a specialist on AI for healthcare at the University of Illinois Urbana-Champaign (UIUC).
“We are trying to create a model that will take the notes that are already being written by primary care physicians and look for unique sets of signs and symptoms that might suggest a patient has an undiagnosed rare disease,” Cross, who is also the leader of the OSF Children’s Innovation Lab at the Jump Trading Simulation & Education Center, said in an OSF press release. “That approach can empower the physician with information regarding those diseases and prompt further testing if they choose to do so.”
The term rare disease is used to describe disorders affecting less than 200,000 people in the U.S. Primary care clinicians are often not familiar with the clinical presentations of rare diseases, due to their rare nature, leading to misdiagnoses or delayed diagnoses.
Urgent need for more accessible tools that may help diagnose rare diseases
Also, while genetic tests are available that can accurately diagnose some of those conditions, these tests are expensive and not readily available in primary care settings, particularly in rural communities. Thus, there’s an urgent need for more accessible tools that may aid in diagnosing rare diseases, which are estimated to affect about 1 in 10 people worldwide.
Despite the complexity of many rare diseases, patients often will share common patterns that are registered in their electronic medical records. These can include symptoms and medical history, as well as demographic features such as race, ethnicity, age, and location.
The new project will use a method called Automated Rare Disease Mining to harness the power of generative AI and machine learning to go through these records and find patterns related to specific rare disorders.
Generative AI is a particular type of algorithm that, rather than simply analyzing and classifying data, can actually be used to create new content in the form of text, images, or videos. Machine learning is a form of artificial intelligence that allows a computer to analyze data and learn from its patterns.
The researchers have spent one year developing robust machine learning models able to extract the relevant information from anonymized electronic medical records. With that data, they’ve built visual maps known as knowledge graphs detailing the relationships between rare disease symptoms and medical conditions.
‘Better visual example of just how complex these diseases are’
“We did this so that we could give people a better visual example of just how complex these diseases are and how they’re all connected,” Cross said. “It’s kind of beautiful when you see the … complexity of all the different symptoms, but the simplicity of the structure. It gives you a sense of just how incredible these new technologies really are in terms of helping us find patterns in the chaos.”
The project is leveraging a supercomputer at UIUC that’s compliant with the federal Health Insurance Portability and Accountability Act, which ensures that healthcare data storage and processing obey strict privacy and security rules.
The project’s next step is to test the models’ predictive abilities. Linking individual patient data with the broad medical knowledge on rare diseases will unveil new patterns and correlations, Cross suggests. Overall, these outcomes are expected to shorten patients’ diagnostic journeys, and perhaps even unveil previously unknown rare disorders.
“As we go along, it is certainly possible with the technology that we’ve created, maybe we’ll start to see patterns of signs and symptoms that don’t have a known diagnosis,” Cross said. “Or maybe there are subtypes of diseases that are out there that really seem to have their own unique progression, and we could also maybe discover those in subsequent phases of the project.”