Researchers create AI algorithm to aid genetic testing decisions
Tool designed to help doctors diagnose rare pediatric diseases like AADC
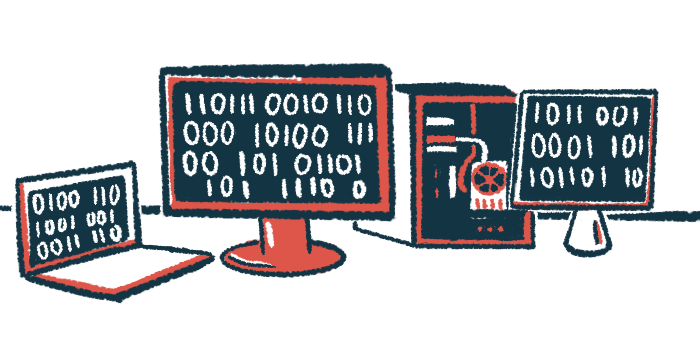
A team of U.S. researchers developed an artificial intelligence or AI-based algorithm to help doctors decide which type of genetic testing is most appropriate for children suspected of having a rare pediatric disease.
The scientists believe their algorithm, dubbed the Phen2Test, will help accelerate the diagnostic odyssey for children living with rare genetic conditions, such as aromatic l-amino acid decarboxylase (AADC) deficiency.
“Patients with rare diseases often experience prolonged diagnostic delays,” the team wrote. “Ordering appropriate genetic tests is crucial yet challenging, especially for general pediatricians without genetic expertise.”
This new AI algorithm “achieved a remarkable performance,” according to the researchers, “aligning closely with decisions made by genetic specialists, … indicating its potential value for general pediatricians to expedite rare disease diagnosis by enhancing genetic test ordering.”
The study, “Phenotype driven molecular genetic test recommendation for diagnosing pediatric rare disorders,” was published in the journal npj Digital Medicine.
AI algorithm compares costs of different testing approaches
AADC deficiency is an extremely rare genetic condition caused by mutations in the DDC gene. Patients experience a range of neurological symptoms that include developmental delays, movement disorders, and behavioral challenges.
Diagnosing rare conditions like AADC deficiency is challenging, as many doctors may not be familiar with their signs and symptoms. But diagnostic delays and misdiagnoses can mean that patients must wait longer to receive possibly life-saving treatments.
Genetic testing is the most definitive way to reach a diagnosis for these rare inherited conditions. Depending on an individual’s clinical presentation, a doctor might test for a specific gene linked to a disease, or run a gene panel to look at several genes that could be associated with the person’s symptoms.
Genetic testing strategies that can screen all the genes in a person’s body at once also are available. But such testing, which includes whole exome sequencing, or ES, and whole genome sequencing, or GS, is expensive to run.
ES and GS both offer a high diagnostic yield, but the results are time-consuming to analyze, and the testing is costly, so they’re often not used until smaller gene panels have proven inconclusive.
However, some newer guidelines suggest that directly using ES or GS as a first-line approach may actually be more efficient and cost-effective in testing for rare diseases. This may especially be true in cases in which a patient’s symptoms are nonspecific — such as developmental delays or congenital abnormalities — and the genetic underpinnings can’t be easily narrowed down.
“Deciding whether to use ES/GS or gene panels as the first test is becoming a crucial and complex, case-by-case decision for clinicians, particularly when there is strong suspicion of a genetic basis for the condition,” the researchers wrote.
They noted that this can be especially challenging for pediatricians who don’t have specialized genetics training.
The goal of the researchers’ Phen2Test was to develop a strategy that could help general pediatricians more easily make testing decisions, thereby speeding the diagnostic process for patients with rare diseases.
Put simply, their algorithm examines patients’ characteristics from their medical records to predict whether an experienced genetic specialist would bypass smaller gene panels in favor of direct ES or GS testing.
Prospective studies still needed to test model in clinical settings
The scientists developed the model using an AI approach called machine learning, in which the algorithm is trained to recognize patterns and make predictions by analyzing large amounts of data.
In this case, the algorithm was trained on more than 1,000 electronic health records from patients younger than age 19 who were seen by geneticists at Columbia University’s Irving Medical Center in New York.
The model, which considered diagnostic billing codes and demographic information, was found to be highly accurate for choosing the right diagnostic test, with a performance similar to that of genetic specialists, according to the researchers.
It also was significantly more accurate than the performance of a general pediatrician who does not routinely order genetic testing, the team noted.
The scientists then validated their findings in separate groups of patients, determining that the model maintained its predictive abilities.
This retrospective study develops and validates an accurate … approach to identify suitable molecular genetic tests needed to diagnose rare pediatric disorders for clinicians with minimal knowledge about genetic tests.
Finally, the team performed a cost-benefit analysis to help understand how using the Phen2Test might be cost-effective relative to other methods of clinical decision-making.
In comparison to a clinical approach wherein a doctor orders a targeted gene panel and progresses later to ES/GS if the results are negative, the analysis estimated that using the Phen2Test to directly select a genetic testing strategy would save $536 per patient “relative to the tiered approach.”
Compared with a strategy in which a doctor directly orders ES/GS, the expected savings in testing costs were $236 per patient.
Overall, “this retrospective study develops and validates an accurate … approach to identify suitable molecular genetic tests needed to diagnose rare pediatric disorders for clinicians with minimal knowledge about genetic tests,” the researchers wrote.
The team noted that a few things remain to be worked out before the system would be able to be used in routine clinical care.
For example, the model was trained using data from resource-rich academic centers. Thus, the generalizability of the model may be limited when applied to lower-resource healthcare systems, such as those in rural areas, where ES/GS are not used as widely, the team noted.
“More studies are warranted to test the effectiveness of this approach prospectively in clinical settings and the generalizability to different [healthcare] systems,” the team concluded.