AI may help detect AADC deficiency in electronic health records
Diagnosing condition requires that at least two of three positive diagnostic tests
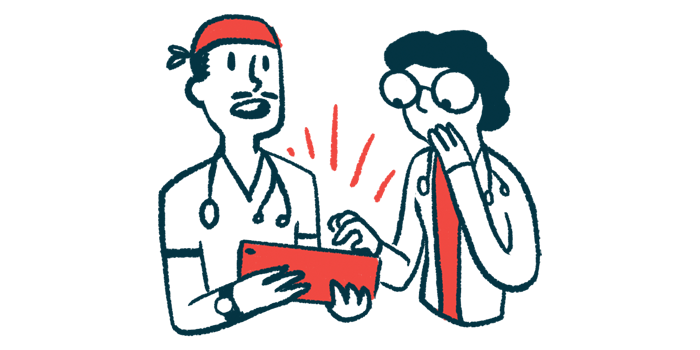
Machine learning, a form of artificial intelligence (AI), was applied to electronic health records to identify young people who may have undiagnosed aromatic l-amino acid decarboxylase (AADC) deficiency, a study reports.
Based on a manual review, nearly 23% of the top-ranked predicted cases were marked for diagnostic testing consideration versus none of the bottom-ranked cases — a statistically significant difference.
The findings should be followed by an expert review of selected patients and potential laboratory testing to confirm the presence of the ultra-rare genetic disease, the researchers said in “Automatically pre-screening patients for the rare disease aromatic L-amino acid decarboxylase deficiency using knowledge engineering, natural language processing, and machine learning on a large EHR population,” which was published in the Journal of the American Medical Informatics Association.
AADC deficiency is caused by mutations in the DDC gene, which lead to faulty or absent AADC, the enzyme responsible for producing certain neurotransmitters, molecules nerve cells use to communicate, including serotonin and dopamine.
The onset of AADC deficiency symptoms typically occurs in infancy with low muscle tone (hypotonia), developmental delays, feeding problems, and oculogyric crises, or periods marked by the involuntary upward deviation of the eyes. Other symptoms may include problems with involuntary bodily processes, or autonomic dysfunction, seizures, and mood disorders. The condition “often presents as a nonspecific neurodevelopmental disorder, particularly when the distinguishing feature of the oculogyric crisis is not recognized,” the researchers wrote. As a result, patients can be misdiagnosed with other neurologic disorders, including cerebral palsy and/or seizures.
Diagnosing AADC deficiency with machine learning
Diagnosing AADC deficiency requires that at least two of three positive diagnostic tests. These include a genetic test to look for DDC gene mutations and a blood test to see if AADC enzyme activity is reduced. The other test requires an invasive spinal tap to measure the levels of neurotransmitter-related molecules in the fluid surrounding the brain and spinal cord.
A possible way to expedite AADC deficiency diagnosis is to use clinical data, particularly those from electronic health records (EHR), coupled with machine learning, which uses algorithms to analyze data, learn from its analyses, and then make a prediction about something.
The goal of the study by researchers at the Oregon Health & Science University (OHSU) was to use EHR data and machine learning to detect patients who may have undiagnosed AADC deficiency. The work was sponsored by PTC Therapeutics, the developer of Upstaza (eladocagene exuparvovec), a one-time gene therapy designed to deliver a healthy copy of the DDC gene to nerve cells. The therapy is approved in the European Union and in the U.K. to treat patients ages 18 months and older. The company expects to submit a regulatory application to the U.S. Food and Drug Administration in the coming months following a meeting in December.
The researchers’ approach was not based on training computers with confirmed AADC deficiency cases, but rather on recognizing symptoms and associated conditions in EHRs that together may suggest an undiagnosed neurotransmitter disorder.
“The goal is to identify cases where a definitive diagnosis is not present in the chart and diagnostic testing for [AADC deficiency] may be indicated,” the researchers wrote.
Developing the dataset
The 10 most important factors associated with AADC deficiency risk in EHRs were selected first. These included oculogyric crisis, movement disorders, mood disturbances, insomnia, feeding issues, hypotonia, epilepsy or seizures, developmental delay, cerebral palsy, and autonomic dysfunction.
The data source was OHSU patients represented in a standards-based data model called Observational Medical Outcomes Partnership Common Data Model. Patients included were those aged 25 or younger with at least two recorded visits to OHSU and at least one pediatric neurology note.
The resulting dataset contained 8,946 patients and 520,473 notes. These were randomly divided into 10 groups of about 850 cases each, called partitions, in which partition 0 was for training and the remaining partitions (1-9) were the blinded test dataset.
Using machine learning, each sentence in the notes was scored for the probability of an AADC deficiency risk factor. All sentence predictions for an individual patient were combined into a single prediction value.
This methodology was applied to the 8,025 patients in the blinded partitions 1-9, then the top-ranked and bottom-ranked 200 patients were identified, and their clinical notes were manually reviewed.
Based on these reviews, 45 (22.5%) of the 200 top-ranked cases were characterized as requiring diagnostic screening. That is, “almost 23% of the top-ranked cases were marked for review by a clinician disease expert and for potential diagnostic testing,” the researchers wrote.
None of the bottom-ranked patients were deemed appropriate for screening. “This result was statistically significant to a high degree,” the researchers wrote. “While review by clinical experts would be the next step, those resources were not available in this study” and “determining an actual diagnosis of [AADC deficiency] will require laboratory testing of patients after pediatric neurologist screening.”
Still, the findings “demonstrated a novel, feasible, generalizable approach for detecting potential undiagnosed cases of rare diseases in large population EHR systems, applied to the specific rare disease of [AADC deficiency],” the researchers wrote. “Future work will enhance the approach to a wider range of diseases, include structured EHR data for patient filtering, and follow up the current research with a detailed clinician review of selected patients.”